In this article, I will discuss Meta’s recent move to test its in-house AI training chip. This initiative aims to reduce reliance on third-party hardware, enhance AI performance, and cut costs.
By developing its own chip, Meta seeks to optimize efficiency for AI models powering platforms like Facebook, Instagram, and WhatsApp.
Introduction
Meta Platforms – which is the parent company of Facebook, Instagram, and WhatsApp – has begun to test its own AI training chip as a part of its strategic goals.
This step is within the broader strategy of Meta to reduce dependence on external chip suppliers and boost the efficiency of the AI processes within the infrastructure of the company.
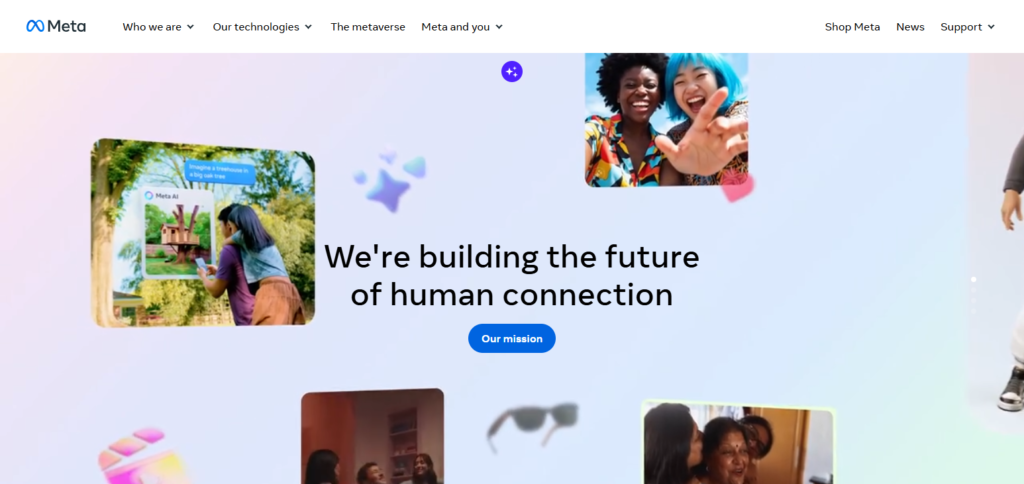
The meta chip is custom built to execute high level AI tasks like working with large language models (LLMS) and recommendation systems.
Key Points Overview
Key Points | Details |
---|---|
Chip Name | Meta Training and Inference Accelerator (MTIA) |
Purpose | AI model training and inference tasks |
Efficiency Gains | Improves performance and reduces costs |
Third-Party Independence | Reduces reliance on Nvidia and AMD |
Current Phase | Early testing stage |
Potential Impact | Boosts AI capabilities across Meta’s apps |
META’s Reason for Chip Development:
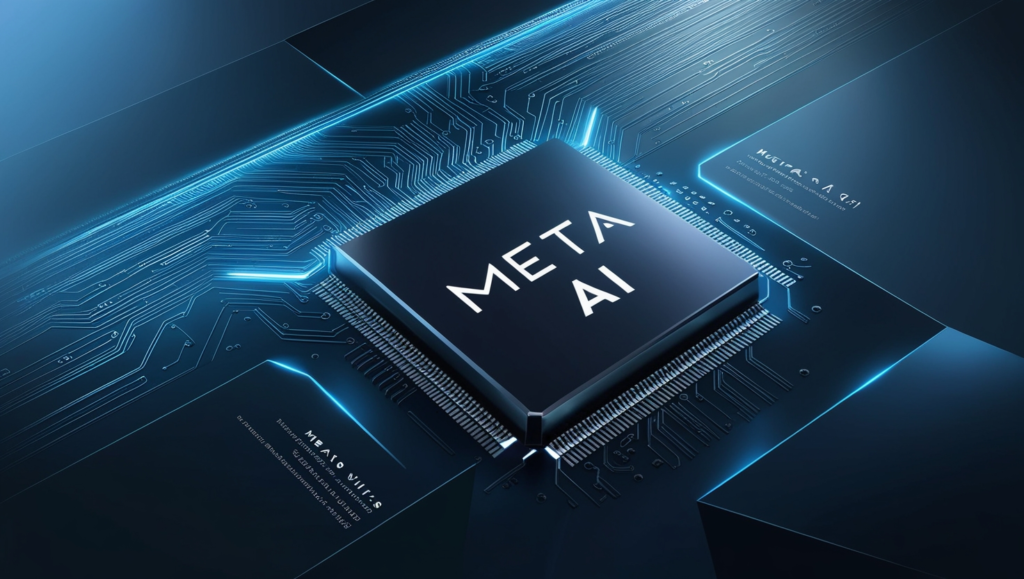
Higher supply constraints and costs because of the reliance on third party hardware, mainly Nvidia, has forced Meta to invest more on AI to improve content recommendations, user experiences, and create advanced AI assistants.
SoMeta seeks to achieve multiple goals by developing its own AI chip, such as:
- Cutting Back Expenditures: Stop using external GPU suppliers.
- Increase Control: Better scalability and performance flexibility.
- Boost Productivity: Internal Requirements Optimization – Design Meta specific AI models.
Features of Meta’s AI Chip
Custom Architecture: The Meta Training and Inference Accelerator (MTIA) supports separate training and inference workloads which makes it flexible for different kinds of AI tasks.
Meta’s AI Chip Specs and Features
Efficiency in architecture
The MTIA Chip has a lens through which it views parallelism that is designed specifically for it. This means that both training and inference workloads can be handled at the same time. This makes sure that the chip can perform numerous AI tasks successfully.
When an AI model is created, it is trained with enormous amounts of data, which is referred to as ‘training’. The step which follows is implementing the model so that it can either make predictions or recommendations, which is called ‘inference’.
If these workloads are optimized, then complex AI applications such as large language models, recommendation systems, and computer vision get better performance with metas larger scale models.
Performance
Increasing efficiency across the board helps to design an economical multi-purpose AI chip, which in turn makes the set challenging to meet power efficiency goals.
Mcs meta’s AI powered multi use chip, meta uses for large scale operations posted lower than expected profits during dismal 22 reporting season when higher spending and lower revenue was expected.
For data centers on the other end, this allows for basic computing without extraordinary expenditure increase getting anywhere near engaging AI operations as meta centers need doing continuously. As a result, performance can be enhanced without a significant increase in the energy expense.
Flexibility
The ability to deploy Meta’s highly efficient chips (MTIA) all over the world adds to its appeal. MTIA can be integrated in the ever-growing network of data centers and power
AI tasks on Facebook, Instagram, and WhatsApp. Flexibility across the ecosystem, even with so much strain and adding load to the system makes it easier to meet targets.
Before, AI performance on Meta platforms would significantly rely on third-party suppliers. However, with the development of Meta’s new AI chip, the company’s overall dependency on external suppliers will further decline
while cost-effectiveness and performance will increase. nbsp; This marks a further progression in Meta’s AI infrastructure development.
Meta Development Goals
High Processing Power: Meant to execute deep learning, recommendation algorithms, and other related AI models on a large scale.
Memory Bandwidth: It has high bandwidth memory (HBM) to increase data retrieval and processing rates.
AI Optimization: Proficient in natural language processing (NLP), computer vision, and recommendation engines.
IT Cost Reduction: Increases the speed of inference by lowering latency, which allows real-time AI prediction and recommendations.
Lower Cost: Saves Meta from purchasing GPU hardware from other companies, cutting costs significantly.
Data Center Integration: Enhances existing infrastructure AI performance and integrates with Meta systems seamlessly.
Impact on Meta’s AI Ambitions
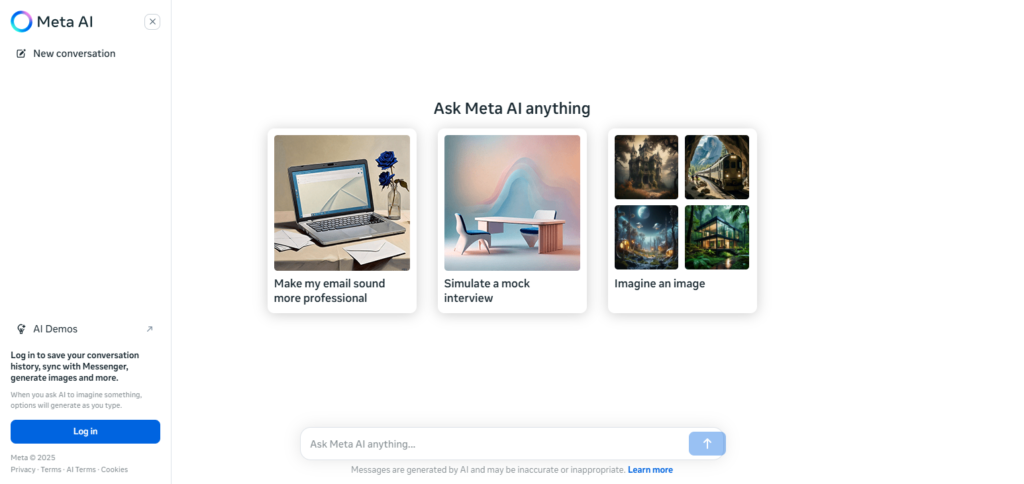
- Lower The Cost and Increase The Speed of Running AI Tasks: Execute advanced algorithms to support large language models (LLMs), as well as sophisticated computation vision models.
- Increase The Level Of Sophistication And Fine-tune AI Components Of Facebook, Instagram, And WhatsApp: Integrate AI capabilities into more advanced features of Facebook, Instagram, and WhatsApp.
- Eliminate Reliance on Outside Vendors: Achieve autonomy from external equipment manufacturers.
Challenges and Future Outlook
Meta’s proprietary AI chip offers advantages; however, it comes with a range of challenges.
1. Battling Industry Giants:
Entering the already competitive AI hardware market dominated by Nvidia and AMD is a challenge for Meta, who is trying to build a reputation in the industry. These companies have established market presence, infrastructure, and customer loyalty.
Matching their performance, reliability, and speed will be difficult on Meta. The chip developed by Meta would need to outperform their competitors in speed, cost, and performance to gain a foothold.
2. Increased Metascope Skepticism:
Still, the efficiency of the chip when deployed at scale is questionable. While internal tests might yield positive results, rolling out the chip across all of Meta’s datacenters comes with its own set of challenges.
Meta will have to ensure that systems work in a stable, repeatable, and efficient manner under heavy AI workloads. The scoping skepticism goes Meta’s dataset growth rate as well; reliability is key. That said, it will take a lot of work on Meta’s side before deploying it.
Future Projection:
If successful, Metas proprietary AI chip scans scan change the game in the Ai infrastructure market.
The custom offering coupled with enabling cloud-based AI services, opens wide-ranging prospects in his proprietary chip technology. Moreover, The firm can use its Meta-derived chip technology beneath branded caps.
Tsai concluded that Meta’s success with the chip would lessen its reliance on external vendors while simultaneously improving its AI skills.
Conclusion
In conclusion Meta’s own AI training chip prototypes indicate a movement towards self-sufficiency in AI hardware. If it goes according to plan, Meta’s AI cost and performance efficiency will improve while strengthening its competitive advantage.
Meta’s goal of minimizing reliance on external vendors is meant to improve Mmeta’s AI performance on Facebook, Instagram, and WhatsApp.