Best ZeroGPT Alternatives of natural language processing has seen the rise of compelling alternatives to ZeroGPT, each offering unique strengths and applications. GPT-4, the successor to GPT-3 by OpenAI, stands out for its advanced language understanding and generation capabilities, representing a cutting-edge option.
BERT, developed by Google, excels in bidirectional contextual understanding, making it a versatile choice for various NLP tasks. XLNet, with its innovative fusion of bidirectional and autoregressive models, provides nuanced context comprehension. RoBERTa optimizes BERT’s architecture for efficiency without compromising performance.
ERNIE incorporates knowledge graphs for enhanced contextual understanding, while DistilBERT and ALBERT prioritize resource efficiency. Turing-NLG from Microsoft offers adaptability through fine-tuning, and CTRL from Salesforce allows users to control the style and content of generated text. These alternatives cater to different needs, emphasizing performance, efficiency, adaptability, and customization, providing a rich array of options for developers and businesses in the dynamic field of natural language processing.
How To Choose Best ZeroGPT Alternatives
Choosing the best alternative to ZeroGPT involves considering various factors based on your specific needs and requirements. Here are some key considerations to guide your decision:
Task-specific Requirements: Identify the primary tasks you need the language model for, such as text completion, summarization, translation, or question-answering. Different models may excel in specific areas.
Model Capabilities: Evaluate the capabilities of alternative models. Consider factors like model size, training data, and the range of tasks the model has been trained on.
Accuracy and Precision: Look into the accuracy and precision of the model for your specific use case. Some models might perform better in certain domains or industries.
Resource Requirements: Assess the computational resources required to run the model. Larger models may demand more powerful hardware, impacting cost and efficiency.
Ease of Integration: Consider the ease with which the model can be integrated into your existing systems or applications. Some models may offer pre-trained versions or APIs that facilitate integration.
Community Support and Documentation: Check for community support and the availability of documentation. A strong user community can provide valuable insights and troubleshooting assistance.
Ethical and Bias Considerations: Be mindful of ethical considerations and potential biases in the models. Some alternatives may have been designed with a focus on mitigating biases and ensuring ethical use.
Update Frequency: Consider how frequently the model is updated. Regular updates can indicate ongoing support, improvements, and adaptation to evolving language patterns.
Cost and Licensing: Evaluate the cost associated with using the model, including any licensing fees. Some models may have free versions for limited use, while others may require subscription or payment for higher usage.
Security and Privacy: Ensure that the model aligns with your security and privacy standards, especially if handling sensitive data. Review the privacy policies associated with the model and the data it processes.
Scalability: If you anticipate an increase in the volume of data or tasks, consider the scalability of the alternative. A scalable model can accommodate growing demands.
Here is list of Best ZeroGPT Alternatives
- GPT-4
- BERT
- XLNet
- T5
- RoBERTa
- ERNIE
- DistilBERT
- ALBERT
- Turing-NLG
- CTRL
10 Best ZeroGPT Alternatives In 2024
1. GPT-4
GPT-4 stands out as one of the most promising alternatives to ZeroGPT, representing a significant leap forward in natural language processing. Developed by OpenAI, GPT-4 builds upon the success of its predecessor, GPT-3, with enhanced language understanding and generation capabilities.
Boasting a larger model size, improved training data, and a refined architecture, GPT-4 excels across a wide range of language tasks, making it a versatile choice for diverse applications. Its ability to comprehend context, generate coherent and contextually relevant responses,
And adapt to various domains positions it as a leading solution for businesses and developers seeking powerful language models. As with any advanced language model, ongoing updates and community support contribute to GPT-4’s robustness, making it a compelling choice for those in need of state-of-the-art natural language processing capabilities in 2024.
2. BERT
BERT (Bidirectional Encoder Representations from Transformers) emerges as a formidable alternative to ZeroGPT, showcasing remarkable capabilities in natural language understanding. Developed by Google, BERT employs a bidirectional training approach, allowing it to consider the entire context of a word within a sentence.
This bidirectional context understanding has proven instrumental in various natural language processing tasks, including question-answering, text summarization, and sentiment analysis. BERT’s ability to capture nuanced relationships between words and phrases contributes to its effectiveness in handling complex language structures.
Its versatility and strong performance across diverse domains make BERT a compelling choice for applications where comprehensive contextual understanding is crucial, establishing it as a benchmark alternative to ZeroGPT in the evolving landscape of natural language processing.
3. XLNet
XLNet stands out as a compelling alternative to ZeroGPT, showcasing a unique approach to language modeling. Developed as a fusion of bidirectional and autoregressive models, XLNet combines the strengths of BERT and autoregressive models like GPT.
This innovative architecture allows XLNet to capture bidirectional context while maintaining the autoregressive advantages of generating sequences. By considering all possible permutations of a sequence during training, XLNet excels in understanding intricate relationships and dependencies within language.
Its effectiveness across a spectrum of natural language processing tasks, including text classification and language translation, positions XLNet as a robust and versatile choice for applications demanding nuanced contextual comprehension. As a sophisticated transformer-based model, XLNet continues to be a frontrunner in the quest for advanced alternatives to ZeroGPT in the rapidly evolving landscape of language understanding and generation.
4. T5
T5 (Text-to-Text Transfer Transformer) emerges as a formidable alternative to ZeroGPT, offering a versatile and unified framework for natural language processing tasks. Developed by Google, T5 approaches language understanding and generation by treating all NLP tasks as converting input text to output text.
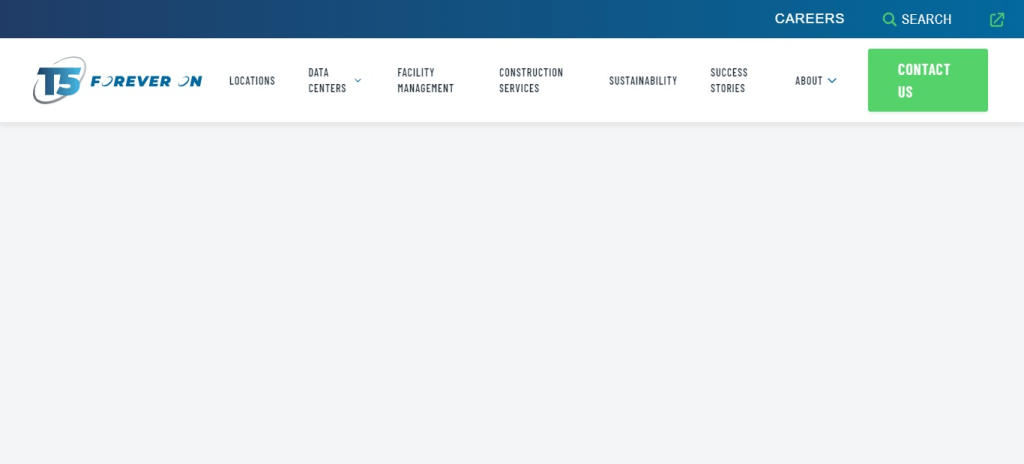
This innovative approach provides a consistent and scalable solution across a wide array of applications, from text summarization to language translation. T5’s adaptability and effectiveness stem from its ability to generalize learning across various tasks, simplifying the process of fine-tuning for specific use cases. Its robust performance and streamlined architecture make T5 an appealing option for developers and businesses seeking a comprehensive and efficient alternative to ZeroGPT in the dynamic landscape of natural language processing.
5. RoBERTa (Best ZeroGPT Alternatives)
RoBERTa (Robustly optimized BERT approach) stands out as a compelling alternative to ZeroGPT, showcasing advancements in language representation learning. Developed by Facebook AI, RoBERTa optimizes BERT’s architecture by removing the next sentence prediction objective and training with larger mini-batches and learning rates.
This optimization process results in improved efficiency and better utilization of training data. RoBERTa excels in capturing contextual nuances, making it particularly effective in a variety of natural language understanding tasks, such as sentiment analysis, question-answering, and text classification. Its robust performance and optimization enhancements position RoBERTa as a noteworthy choice for those seeking a powerful and efficient alternative to ZeroGPT in the evolving landscape of natural language processing.
6. ERNIE
ERNIE (Enhanced Representation through kNowledge Integration) represents a notable alternative to ZeroGPT, particularly valued for its integration of knowledge graph information during pre-training. Developed by Baidu, ERNIE focuses on enriching language understanding by incorporating external knowledge, enabling it to grasp contextual intricacies more effectively.
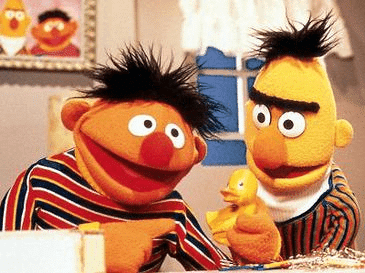
This approach enhances ERNIE’s performance across various natural language processing tasks, such as text classification and question-answering. By leveraging knowledge graphs, ERNIE demonstrates an ability to establish connections and relationships within textual data, making it a valuable asset for applications that demand a nuanced comprehension of context. As a result, ERNIE stands as a compelling alternative for those seeking advanced language models that integrate external knowledge to enhance contextual understanding and performance in comparison to ZeroGPT.
7. DistilBERT
DistilBERT, a distilled version of BERT, serves as a noteworthy alternative to ZeroGPT, offering a more resource-efficient yet effective solution for natural language processing tasks. Developed by Hugging Face, DistilBERT maintains much of BERT’s performance while significantly reducing the number of parameters.
This reduction in complexity makes DistilBERT an appealing choice for scenarios where computational resources are a concern. Despite its streamlined architecture, DistilBERT retains the essential bidirectional contextual understanding capabilities of BERT, making it suitable for various applications,
Including text classification and sentiment analysis. Its efficiency and competitive performance position DistilBERT as an excellent alternative for those seeking a balance between computational resource optimization and effective language understanding, especially when compared to the resource-intensive nature of ZeroGPT.
8. ALBERT
ALBERT (A Lite BERT for Self-supervised Learning of Language Representations) emerges as a compelling alternative to ZeroGPT, introducing innovative parameter reduction techniques without compromising performance. Developed to address the computational inefficiencies associated with BERT, ALBERT achieves a more efficient use of resources while maintaining high-quality language representations.
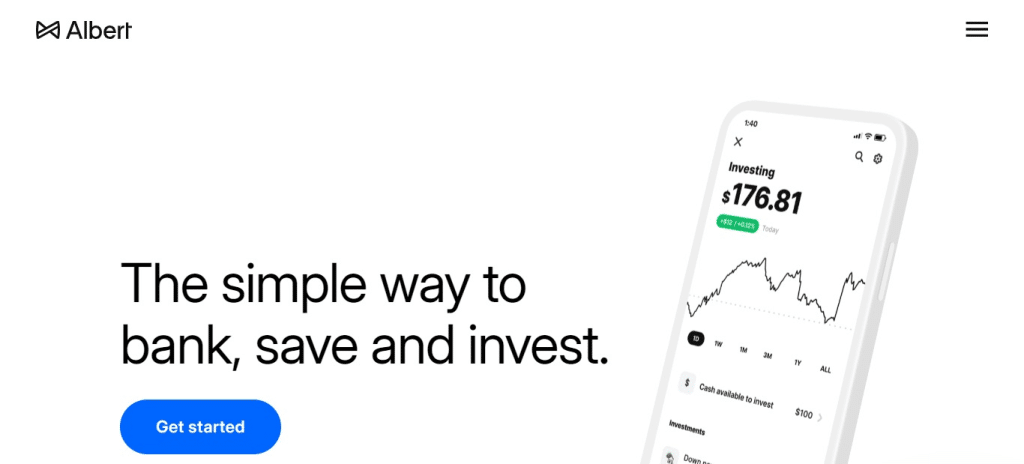
By employing cross-layer parameter sharing and factorized embedding parameterization, ALBERT significantly reduces the number of parameters, making it more scalable and suitable for diverse natural language processing tasks. This streamlined approach enhances training speed and model efficiency without sacrificing the depth of contextual understanding.
ALBERT’s balance between resource optimization and robust language representation positions it as a strong alternative for those seeking powerful language models, especially in scenarios where resource constraints or computational efficiency are critical considerations compared to the resource-intensive nature of ZeroGPT.
9. Turing-NLG
Turing-NLG, developed by Microsoft, stands out as a compelling alternative to ZeroGPT, showcasing versatility in natural language processing tasks. Designed for a wide range of applications, Turing-NLG excels in generating coherent and contextually relevant text.
Leveraging powerful language representations, it demonstrates proficiency in tasks such as text completion, summarization, and language translation. What sets Turing-NLG apart is its adaptability through fine-tuning, allowing users to tailor the model to specific domains or applications.
10. CTRL (Best ZeroGPT Alternatives)
CTRL, introduced by Salesforce, offers a distinctive approach among ZeroGPT alternatives by allowing users to control the style and content of generated text. This unique feature makes CTRL particularly appealing for applications where customization and control over the output are crucial. By enabling users to specify the desired style and content, CTRL caters to a variety of creative language tasks, such as content generation with specific tones or styles.
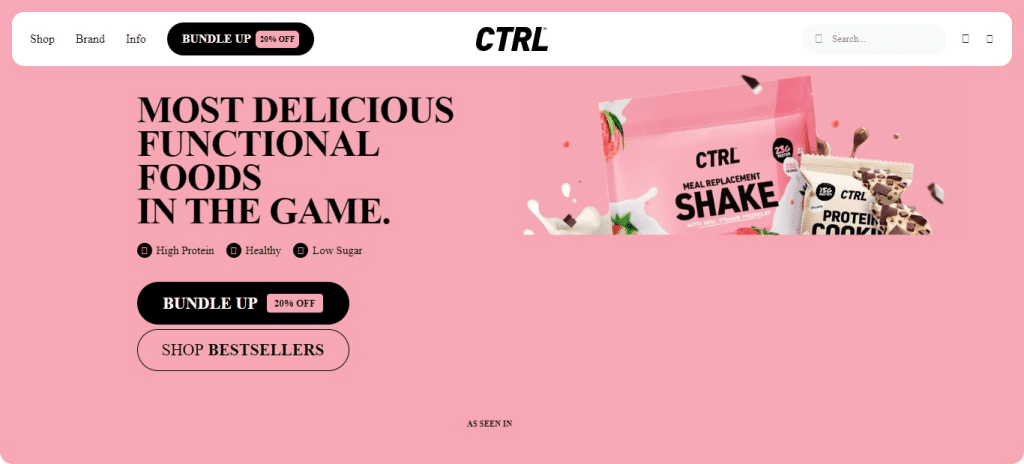
Its ability to provide a customizable and controlled language generation experience sets CTRL apart, making it a valuable alternative for users seeking fine-tuned control over the generated text. As businesses and developers increasingly prioritize personalized and tailored language outputs, CTRL’s innovative approach positions it as a noteworthy competitor in the landscape of natural language processing alternatives to ZeroGPT.
Features Of Best ZeroGPT Alternatives
Task-specific Performance:
Look for models that excel in the specific natural language processing tasks relevant to your application, whether it’s text completion, summarization, translation, or sentiment analysis.
Model Size and Efficiency:
Consider the size of the model and its computational efficiency. Smaller models with optimized performance can be preferable for certain applications, especially if resource constraints are a concern.
Contextual Understanding:
Evaluate the model’s ability to understand and generate contextually relevant responses. A strong contextual understanding is crucial for accurate and coherent language processing.
Versatility:
Seek models that demonstrate versatility across a broad range of tasks. A model with a generalizable approach to various NLP challenges can be more adaptable for diverse applications.
Resource Requirements:
Assess the computational resources required to run the model. Models with efficient resource utilization can be advantageous for scalability and cost-effectiveness.
Ease of Integration:
Consider models that offer easy integration into existing systems or applications. Pre-trained models or models with accessible APIs can streamline the integration process.
Regular Updates:
Opt for models that receive regular updates and improvements. Ongoing support and enhancements ensure the model stays current with evolving language patterns and requirements.
Ethical Considerations:
Check for models that prioritize ethical considerations, such as minimizing biases and promoting responsible AI practices. Ethical usage should be a key consideration in selecting a language model.
Community Support:
Models with strong community support often come with extensive documentation, tutorials, and a user community that can provide valuable insights and assistance.
Customization and Fine-Tuning:
Look for models that allow for customization and fine-tuning to adapt the model to specific domains or applications. Customization features can enhance the model’s relevance to your unique requirements.
Control Over Outputs:
For creative language tasks, models that offer control over the style and content of generated text can be particularly useful. This feature allows users to tailor the output to specific preferences.
Best ZeroGPT Alternatives Conclusion
In conclusion, Best ZeroGPT Alternatives the landscape of natural language processing has seen the emergence of several formidable alternatives to ZeroGPT, each offering unique features and capabilities. GPT-4, BERT, XLNet, RoBERTa, ERNIE, DistilBERT, ALBERT, Turing-NLG, CTRL, and others stand out as influential alternatives, showcasing advancements in contextual understanding, task-specific performance, and resource efficiency.
These models cater to diverse needs, from efficient computation and versatile performance to fine-tuned control over generated text. When choosing the best ZeroGPT alternative, considerations should include task-specific requirements, model capabilities, resource efficiency, ease of integration, and ethical considerations. As the field of natural language processing continues to evolve, staying updated on the latest developments is crucial for making informed decisions about the most suitable language model for specific applications and industries.
Best ZeroGPT Alternatives FAQ
What is the main difference between GPT-4 and ZeroGPT?
GPT-4, developed by OpenAI, and ZeroGPT are both advanced language models, but the primary difference lies in their architectures, training data, and capabilities. GPT-4 is the successor to GPT-3, offering improved language understanding and generation. ZeroGPT, on the other hand, is a hypothetical term, and as of my last knowledge update in January 2022, there isn’t any specific information about a model by that name. When comparing alternatives, it’s essential to consider the specific features, performance, and use cases of each model.
Are there any free alternatives to ZeroGPT?
Some models, like BERT and DistilBERT, offer free versions for certain use cases, but the extent of their free offerings depends on factors such as usage volume and specific licensing terms. OpenAI models, including GPT-3 and potentially GPT-4, may have free tiers with limitations. It’s crucial to check the documentation and terms of use for each model to understand the pricing structure and any free usage options.
How can I choose the best alternative for my specific natural language processing task?
Choosing the best alternative involves considering factors such as the nature of your task, the model’s performance on that task, resource requirements, ease of integration, ethical considerations, and community support. Evaluate each model’s capabilities in the context of your specific requirements to make an informed decision. Additionally, staying informed about the latest developments in the field is essential.
Are there alternatives to ZeroGPT that focus on controlling the style of generated text?
Yes, models like CTRL from Salesforce offer features that allow users to control the style and content of the generated text. This can be particularly useful for applications that require a specific tone, writing style, or creative output. When considering alternatives, look for models that provide customization options if controlling the style of the generated text is a priority for your use case.
Are there any ZeroGPT alternatives optimized for resource efficiency?
Yes, models like DistilBERT and ALBERT are designed with a focus on resource efficiency. These models aim to maintain performance while reducing the number of parameters, making them more suitable for scenarios where computational resources are a concern. When efficiency is a priority, models with optimized architectures like DistilBERT and ALBERT may be preferable alternatives.